Obtained nsSNPs
The largest SNP database, dbSNP, identified 215 coding synonymous, 290 in the intron region, 68 in the 5’UTR, 1035 in the 3’UTR, 353 nonsynonymous SNPs, and the remaining SNPs (splice sites = 7, frameshift = 43, and nonsense = 32). From this database, 2,063 SNPs were retrieved in total. From the entire list, nonsynonymous SNPs were chosen. Ensembl genome browser 112 was also used for missense SNP validation, which provided 308 nsSNPs as missense. These nsSNPs are carefully evaluated after duplicates are removed. The retrieved SNPs are shown in Fig. 2.
Shows the distribution of various SNP types within the GPx1 gene. The graph depicts the percentage of coding synonymous (orange), coding nonsynonymous (blue), intron region (green), 5’ UTR (yellow), 3’ UTR (black), and other types of SNPs (silver) associated with GPx1.
Identification and screening of damaging nsSNPs
Eight distinguished bioinformatics methods were employed to analyze all nsSNPs obtained from dbSNP to investigate potential implications for the structure and function of the GPx1 protein. Additionally, the NCBI provided the GPx1 protein sequence (ID: NP_000572.2). SIFT, Polyphene-2, Meta-SNP, PhD-SNP, CADD, Panther, SNAP, and Mutation Assessor were among the in-silico techniques used. Replacements are classified as “deleterious” by SIFT if the score (TI = Total Index) is less than 0.05 and as “tolerated” if it is greater than 0.0530,72. SIFT results showed that 162 nsSNPs had negative effects. The likelihood of replacement-related harm is indicated by the PolyPhen-2 score, where values close to one indicate a higher risk of injury73. Polyphene-2 indicates that 145 nsSNPs might be harmful. Such meta-SNP tools aggregate predictions from multiple methods based on machine learning, statistical research, or a combination of both28. 54 nsSNPs were shown to be possibly pathogenic. To determine whether a certain SNP is likely to have an impact on protein function, PhD-SNP uses a Support Vector Machine that has been trained on features derived from input data74. A comprehensive prediction showed that 63 nsSNPs are sick. In addition, the PANTHER (Position-Specific Evolutionary Preservation) tool employs a revolutionary method that includes multiple sequence alignment, computation of substitution, phylogenetic tree construction, and protein homolog discovery. Evolutionary Conservation Score Specific to Position35. After review, it was found that 122 variations are dangerous. Additionally, we employed SNAP (Screening for Non-Acceptable Polymorphisms) tools, which classify SNPs as functional or non-functional based on an algorithm based on neural networks75. It identified 77 variations as dangerous. Similarly, based on its prediction value—a high score indicates deletion, and vice versa—CADD classifies an SNP as harmful or benign76. 42 nsSNPs were found by CADD to be potentially disease-causing. Ultimately, Mutation Assessor77 was used to evaluate the alterations. MutationAssessor identified forty-one extremely dangerous mutations. Figure 3 shows every result produced by the tool. Of the eight approaches, 14 nsSNPs with negative predictions were selected. Since these 14 nsSNPs are thought to be the most dangerous, they were selected for further investigation.
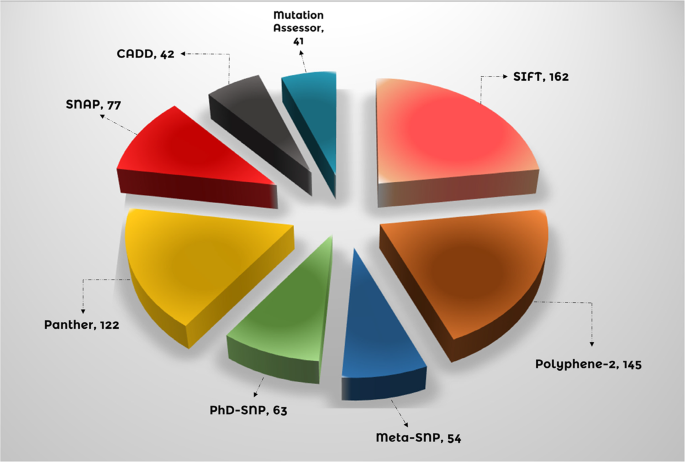
Compares the predictive capabilities of eight bioinformatics tools for identifying potentially damaging nsSNPs in GPx1. The number of nsSNPs, detected by each tool, including Meta-SNP (54), PhD-SNP (63), CADD (42), PolyPhen-2 (145), SIFT (162), SNAP (77), Mutation Assessor (41), and Panther (122), are explained in the form of a pie chart.
MutPred assessment for functional and structural changes
The 14 nsSNPs that were chosen were examined using the MutPred 1.2 server. Table 1 presents the likelihood scores of the findings that were returned. Modifications to the transmembrane protein, ordered interface, catalytic site, relative solvent accessibility, allosteric site, GPI-anchor amidation, N-linked glycosylation, metal binding, and strand were among the predicted structural and functional effects of the server. Data on the previously described attributes, including p-values, are provided in Table 1. Based on a manual criterion of more than 0.5, twelve of the fourteen nsSNPs were likely to change the structure or function of proteins. We investigated these 12 nsSNPs in more detail.
GPx1 stability assessment
I-Mutant 2.0 was used to predict the GPx1 protein stability for 12 nsSNPs with amino acid changes at both 25oC and 37oC temperatures. Each of the chosen nsSNPs was delivered separately, as shown in Table 2, and stability was adjusted using RI values ranging from 0 to 10. Except for one (rs200311870), every single nsSNP that was reported showed a decline in stability. Further research is necessary as this finding suggests that these 11 nsSNPs may have a stronger detrimental effect on the GPx1 protein by reducing its stability.
Evolutionary conservation of GPx1 protein
Understanding evolution is essential for researchers since mutations can affect a person’s health78. The conservation profile of the GPx1 amino acid residues was assessed using DeepREx-WS to anticipate the possible effects of the 11 nsSNPs. The results are shown in Table 3 as a structural diagram of the GPx1 protein. The positions of the 11 nsSNPs found further stimulated our interest, even though the data revealed information on every amino acid in GPx1, with 55.67% of residues exposed and 44.33% hidden. With low conservation < 0.16 and high conservation > = 0.16, the conservation criterion was automatically changed to 0.16. Only four residues—P124, G119, N118, and G37—are predicted by DeepREx-WS to be highly functional, exposed, and conserved. The other largely intact and buried structure remains are likely to be L168, F167, F126, P77, and G75. Because of their poor conservation scores, two residues—W152 and F128—were eliminated. The conservation ratings for every nsSNP that is supplied are shown in Table 3. The function and structure of the GPx1 protein were most negatively impacted by all nsSNPs found in highly conserved regions, according to these results.
Structural modeling of GPx1 and its mutants
To predict changes in the GPx1 protein, the nine most detrimental nsSNPs were chosen, and 3D models of each mutant were created using the Robetta web application. Each nsSNP substitution in the GPx1 protein sequence was carried out separately in the original protein sequence (NCBI ID: NP_000572.2) in order to create mutant protein 3D structures. After that, Robetta received these structures and produced 3D structural predictions. For every mutant model, the RMSD and TM scores were calculated using TM-Align. In wild and mutant models, the average distance between the α-carbon backbones is measured by RMSD, whereas topological similarity is assessed by TM-score. Greater structural divergence between the mutant and the wild type is reflected in greater RMSD values. With RMSD values of 1.48Å, 1.46Å, and 1.45Å, respectively, the mutants L168Q (rs373838463), F167S (rs2107818892), and G75C (rs763687242) exhibited the highest values. The other nsSNPs with lower variance were N118Y (1.41Å RMSD), F126S (1.40Å RMSD), P124L (1.40Å RMSD), G119S (1.40Å RMSD), G37R (1.38Å RMSD), and P77R (136.Å RMSD). The RMSD values and the TM scores are displayed in Table 4. A manual cutoff point of > = 1.45 was set. Three nsSNPs (L168Q, F167S, and G75C) with the highest RMSD values, along with their wild type GPx1, were selected for remodeling using alpha-fold-2. Using Pymol 2.0, Fig. 4 illustrates the three mutations superimposed on top of the wild-type GPx1 protein.
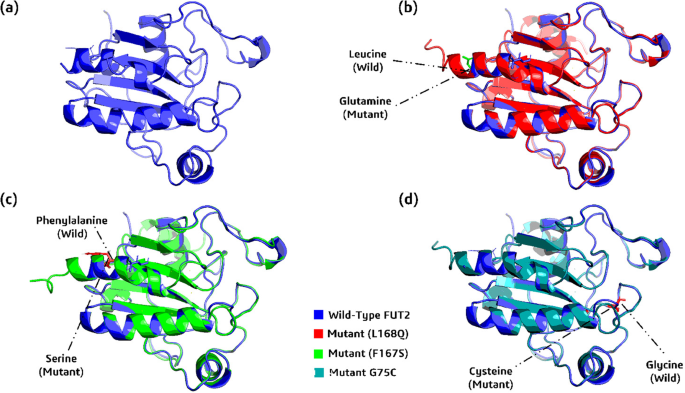
(a) 3D structure of wild_type protein, GPx1 (b) Wild_type GPx1 (blue), along with its superimposed mutant L168Q (red) (c) wild_type GPx1 (blue), along with its superimposed mutant F167S (green), and (d) wild_type GPx1 (blue), along with its superimposed mutant G75C (cyan).
Validation of 3-Dimensional models
After having the modeled 3D structures, MolProbity, QMEAN and SAVES server, were employed to compare the three models against the wild-type GPx1. Qmean demonstrated highest structural accuracy as the scores for G75C, F167S, L168Q and wild-type were 0.85, 0.85, 0.86 and 0.80, respectively. Likewise, MolProbity provided satisfactory outcomes for the wild-type GPx1 and modified modeled proteins. The highest ERRAT scores were exhibited by the mutant and wild-type GPx1 genes: wild_type = 94.94, F167S = 91.98, G75C = 91.94, and L168Q = 91.44. These results indicated the best accuracy and highest quality of modeled structures. A Ramachandran map including the Psi and Psi degrees of the pertinent structures of all GPx1 mutants and wild type has shown in Fig. 5.
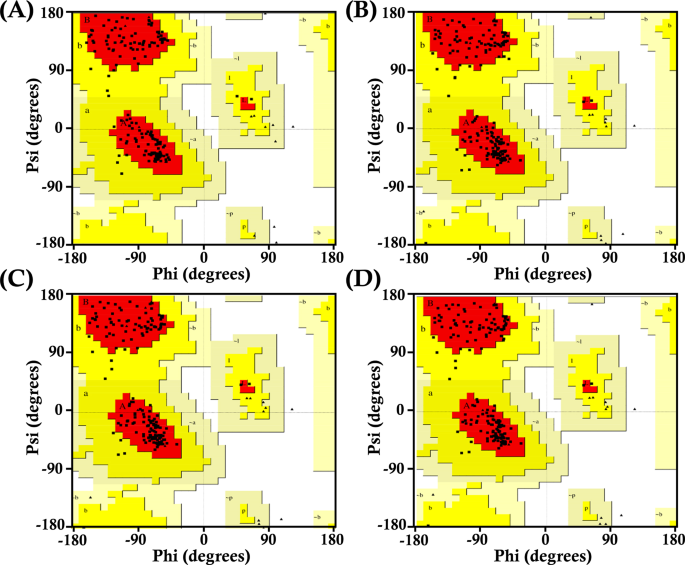
Presents Ramachandran plots for wild-type GPx1 and its mutant variants. These plots visualize the distribution of phi and psi dihedral angles, providing insights into protein conformation (a) Ramachandran plot of wild_type GPx1 (b) Ramachandran plot of L168Q (c) Ramachandran plot of mutant F167S (d) Ramachandran plot of mutant G75C.
Molecular docking analysis
To assess the binding affinity and way of interaction between GPx1 mutants and TRAF2 protein, we docked them together. Using a computational tool called ClusPro v2.0, we simulated this interaction, generating 10 different models for each of the mutant-receptor complex, along with the wild type, GPx1. Among these models, 1 model from each complex was selected. Wild type complex with TRAF2 stood out with the lowest energy (-840.3) and the highest cluster members (98), suggesting a stable and favorable binding between them. Following this, F167S-TRAF2 complex showed binding energy (-758.1) with 69 cluster members, as well as L168Q-TRAF2 complex exhibited comparably low binding affinity (-744.3) and cluster member (63). Among all, G75C showed the lowest binding affinity (-683.9), with cluster members (88). These results (Table 5) demonstrated that G75C, followed by F167S and L168Q, has the lowest binding affinity with TRAF2 and highest possible chances to disruption of connection between them, concluding these mutants as cause of diverse cancers progression.
Additionally, we utilized HADDOCK 2.4 to re-dock all the complexes for validating the docking results of ClusPro. From the total of ten output complexes, the best complex for each mutant and wild-type was selected, based on their highest binding affinity. All of the mutants exhibited lowest binding affinity with the TRAF-2 receptor, as compared to the wild-type (Table 6). The binding affinities of G75C, F167S, and L168Q were − 94.9 ± 3.2, -89.3 ± 4.7, and − 95.1 ± 3.9, respectively, while wild-type complex with TRAF2 showed higher binding affinity as 110.8 ± 3.7. These results validated the output of ClusPro and indicated the possible binding interruption in GPx1-TRAF2 binding due to mutations.
Further analysis using PDBsum revealed a network of interactions within the complexes. We evaluated results based on three interactions; Non-bonded contacts that contribute to the overall attraction; Salt bridges that are specific interactions involving charged atoms, further strengthening the binding; and hydrogen bonds that create precise connections between the molecules, like tiny bridges. G75C mutant showed least interactions with the TRAF2 receptor as containing only 8 hydrogen bonds, and 71 non-bonded contacts. Following this, L168Q mutant also showed low interactions (as compared to wild type) by forming 9 hydrogen bonds and 96 non-bonded contacts. Likewise, F167S mutant showed less interactions as 8 hydrogen bonds, 123 non-bonded contacts and 2 salt bridges. Finally, wild type GPx1 complexed with TRAF2 provided highest interactions as forming 10 hydrogen bonds, 124 non-bonded contacts and 2 salt bridges. The results are shown in Fig. 6. These findings suggest that G75C mutant have lowest interactions with TRAF2, followed by mutant F167S, L168Q and wild type. Thus, it is potentially more likely the cause of diverse cancers’ progression.
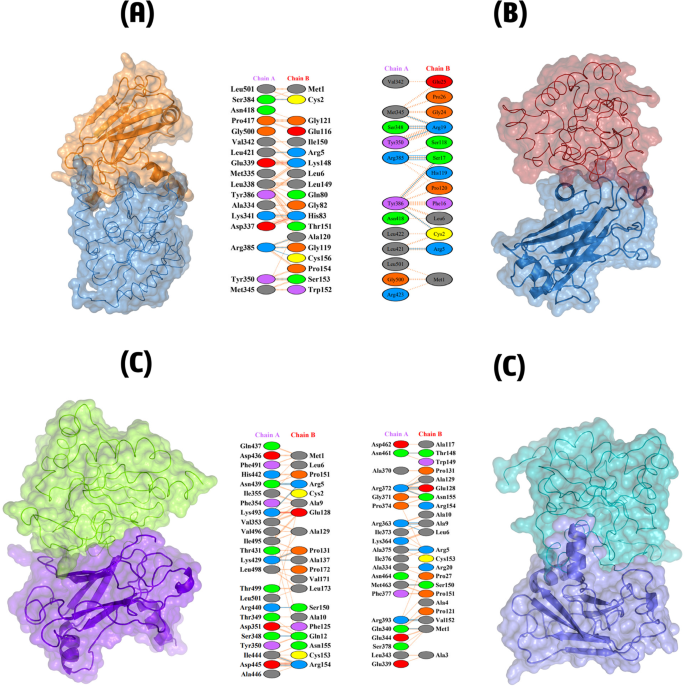
Depicts the 3D structures and interaction networks of the wild-type GPx1-TRAF2 complex and its mutant variants (A) Shows the wild-type GPx1-TRAF2 complex, with the interacting residues highlighted and labeled, (B) Presents the mutant G75C-TRAF2 complex, highlighting the interacting residue, (C) Displays the mutant F167S-TRAF2 complex, focusing on the interacting residues and potential alterations in protein-protein interactions, and (D) Depicts the mutant L168Q-TRAF2 complex, highlighting the interacting residue and any changes in the interaction network.
Molecular dynamic simulation analysis
Compared to the wild type, the G75C, F167S, and L168Q mutants exhibited less stable interactions with the TRAF2 protein (PDB ID: 1CA4). We investigated the time-dependent alterations of these protein-protein complexes over 200 ns using molecular dynamics (MD) simulations. The degree of degenerative alterations and dynamic behavior of the complexes were assessed using specific metrics, including root mean square deviation (RMSD), root mean square fluctuation (RMSF), hydrogen bond analysis, and radius of gyration (RoG). MD simulations were performed on the receptor TRAF2, its three mutants, and the wild type to determine their RMSD, RMSF, hydrogen bond interactions, and radius of gyration. These analyses provided insights into the structural dynamics of the protein-protein complexes over the 200 ns simulation period. The results indicated significant differences in the stability and dynamics of the mutants compared to the wild type, highlighting the impact of these mutations on TRAF2 interaction stability.
Root mean square deviation (RMSD) analysis
To enhance the therapeutic potential of proteins, it is essential to assess their binding stability through molecular simulations. This stability influences the extent to which proteins interact with one another and is crucial for the molecular optimization of novel proteins, enabling accurate evaluation of potential targets79. In this study, we examined binding stability by analyzing simulated trajectories and computing the root mean square deviation (RMSD) over time. We evaluated and validated the stability of each optimized hit, with a particular focus on three selected mutants compared to the wild-type GPx1. Analysis revealed inconsistency in the stability of these mutants. The wild-type GPx1-TRAF2 complex exhibited the highest stability, maintaining an average RMSD between 2Å and 3Å throughout the entire 200 ns simulation (Fig. 7A). In contrast, the F167S-TRAF2 complex displayed the lowest stability, with its RMSD value increasing to 11Å during the first 150 ns, followed by a sudden decline towards the end (Fig. 7B). Similarly, the L168Q-TRAF2 complex showed continuous instability, with its RMSD increasing up to 7.5Å over the entire 200 ns simulation (Fig. 7C). The G75C-TRAF2 complex also exhibited higher deviations, reaching up to 6Å (Fig. 7D). Although this complex demonstrated some degree of stability, its RMSD remained higher than that of the wild type. Overall, the RMSD results indicated that all three mutants displayed greater instability compared to the wild type, potentially disrupting their interactions with TRAF2 and leading to GPx1 overexpression.
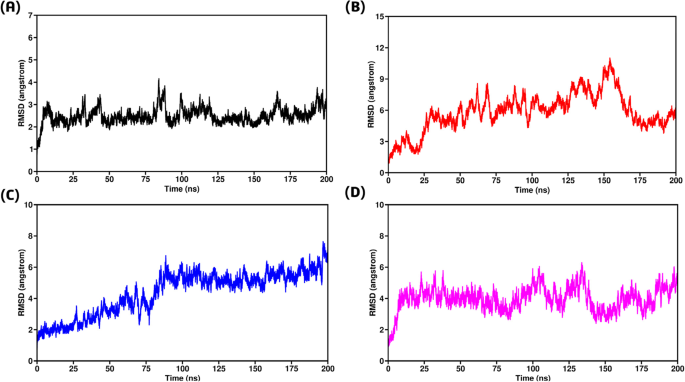
(A) Root Mean Square Deviation of wild type-TRAF2 complex (B) Root Mean Square Deviation of F167S-TRAF2 complex (C) Representing Root Mean Square Deviation of L168Q-TRAF2 complex (D) Root Mean Square Deviation of G75C-TRAF2 complex.
Root mean square fluctuation (RMSF) analysis
Root Mean Square Fluctuation (RMSF) analysis was conducted on individual amino acids to better understand the stability of TRAF2 active site residues during protein interaction80. This investigation spanned the entire 200 ns Molecular Dynamics (MD) trajectory, as depicted in Fig. 8. RMSF values were calculated for all mutations, including the wild type, revealing unique average RMSF values for each system. The wild-type GPx1-TRAF2 complex exhibited the least fluctuations, maintaining stability with RMSF values around 1.5 Å, except for some initial residues (1–5), which indicated the start of the reaction (Fig. 8A). In contrast, the F167S-TRAF2 complex showed the highest fluctuations, with an initial residual decline starting from 9.5 Å. The remaining residues fluctuated between 3 Å to 6 Å, indicating significant instability (Fig. 8B). Similarly, the L168Q-TRAF2 complex displayed fluctuations, with RMSF values ranging from 2 Å to 5 Å throughout, peaking suddenly to 12 Å at the end (Fig. 8C). The G75C-TRAF2 complex demonstrated fewer fluctuations compared to the other mutants but still showed lower stability than the wild type. It began with an initial RMSF of 11 Å for the first 6 residues, followed by less fluctuation between 1 Å to 3 Å, and then a sudden increase to 5.5 Å towards the end (Fig. 8D). Overall, the RMSF results indicated that the wild-type residues remained more stable than those of the mutants throughout the 200 ns simulation. This suggests that the mutations disturbed the interaction with TRAF2, leading to GPx1 overexpression.
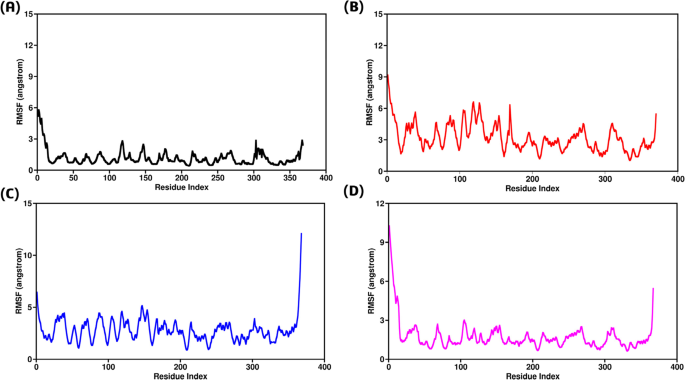
(A) Root Mean Square Fluctuation of wild type-TRAF2 complex (B) Root Mean Square Fluctuation of F167S-TRAF2 complex (C) Representing Root Mean Square Fluctuation of L168Q-TRAF2 complex (D) Root Mean Square Fluctuation of G75C-TRAF2 complex.
Radius of gyration (RoG) analysis
To gain a better understanding of the dynamics and binding/unbinding processes during the simulation, we assessed the structural compactness of each complex under equilibrium conditions. This was achieved by estimating the radius of gyration (Rg) as a function of time81. For the wild type-TRAF2 complex, the Rg values remained consistently low, ranging from 35.7 Å to 36 Å, indicating the highest compactness among all complexes (Fig. 9A). Despite the RMSD and RMSF results, the F167S-TRAF2 complex also exhibited good compactness, with Rg values around 37.2 Å, although slightly higher than the wild type (Fig. 9B). In contrast, the L168Q-TRAF2 complex displayed the highest Rg values, between 40.2 Å and 40.4 Å, suggesting the lowest structural compactness among the complexes studied (Fig. 9C). Similarly, the G75C-TRAF2 complex demonstrated behavior akin to the F167S-TRAF2 complex, with stable Rg values ranging from 37.3 Å to 37.4 Å up to 175 ns, followed by a brief increase towards the end of the simulation (Fig. 9D). Overall, the Rg results indicated that all complexes exhibited similar behavior in terms of structural compactness, except for the L168Q-TRAF2 complex, which had notably higher Rg values.
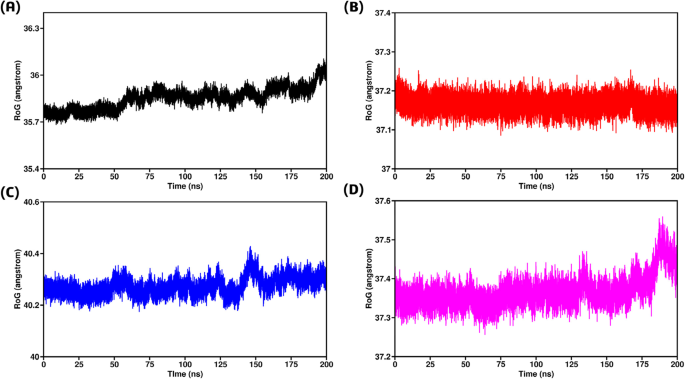
(A) Radius of Gyration between wild type-TRAF2 complex (B) Radius of Gyration between F167S-TRAF2 complex (C) Radius of Gyration between L168Q-TRAF2 complex (D) Radius of Gyration between G75C-TRAF2 complex.
Hydrogen bond analysis
Macromolecular interactions, specifically the bonds between two or more protein molecules, are significantly influenced by hydrogen-hydrophobic interactions at the interface82. To evaluate the systems at the atomic level, we determined the total number of hydrogen bonds formed in each complex using specific criteria: a maximum distance of 0.35 nm between the donor and acceptor atoms, and an angle of 30 degrees between the hydrogen donor and acceptor. These conditions ensured the accurate identification of hydrogen bonds, which are crucial for maintaining the secondary structure of protein-protein complexes83. A time-dependent analysis of hydrogen bonding, as illustrated in Fig. 10, revealed that the three mutant complexes with TRAF2 exhibited weak hydrogen bonding networks compared to the wild type GPx1. All the three mutant complexes displayed a less number of hydrogen bonds (Fig. 10). This suggests that the mutants have a weaker attraction to the TRAF2 receptor compared to the wild type. Consequently, the weaker interactions in the mutant complexes may lead to disturbed binding, potentially causing overexpression of the proteins.
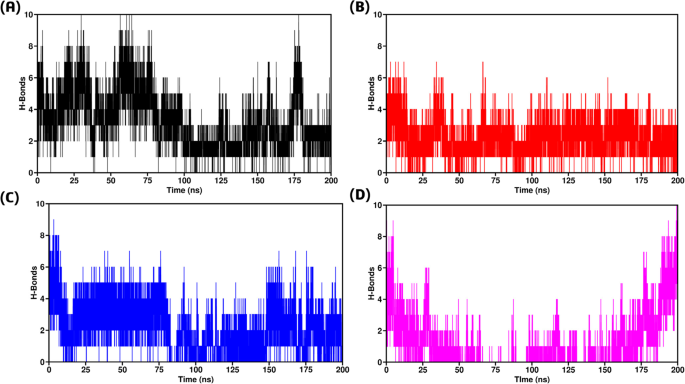
(A) H-bonds between wild type-TRAF2 complex (B) H-bonds between F167S-TRAF2 complex (C) H-bonds between L168Q-TRAF2 complex (D) H-bonds between G75C-TRAF2 complex.
Predicted PTMs (post-transcriptional modifications)
Methylation
GPS-MSP 1.0 identified that none of the GPx1 sites would be methylate.
Phosphorylation
GPS 6.0 and NetPhos 3.1 were used to estimate the phosphorylation sites of GPx1, as shown in Fig. 11. Thirteen residues (Ser:08, Thr:03, Tyr:02) may be phosphorylated, according to NetPhos 3.1 assumptions. Six residues (Ser:02 and Tyr:04) were identified by GPS 6.0 as possibly phosphorylated. In a highly conserved or dangerous nsSNP region, there isn’t a predicted residue that needs to be phosphorylated.
Ubiquitination
GPS-Uber and RUBI were the two websites used in the building of the ubiquity prediction. We estimated that three of the six lysines at locations 38, 88, and 97 would be ubiquitinated using GPS-Uber data. Two of the six lysine residues were predicted by RUBI to be ubiquitinated. An extremely conserved or dangerous nsSNP region is anticipated to have no residues. 16.74% of lysines are thought to malfunction since 33.3% of all lysines are ubiquitinated.
Glycosylation
To determine which glycosylation sites appeared most likely, NetOGlyc4.0 was employed. The wild-type GPx1 protein was glycosylated at positions 15, 20, 137, 145, and 151, with corresponding scores of 0.63, 0.85, 0.76, 0.55, and 0.59. These areas are probably glycosylated.
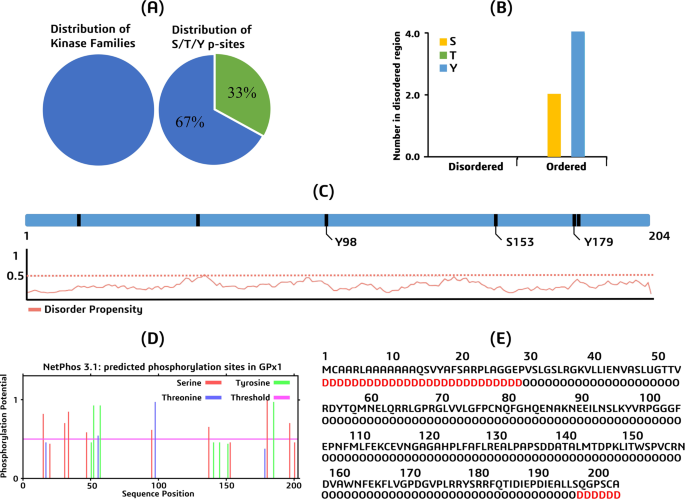
Presents the results of in-silico predictions for potential phosphorylation and ubiquitination sites within the GPx1 protein (a) A pie chart showing the distribution of kinase families predicted to phosphorylate GPx1 residues. The pie chart is divided into two segments: “S/T/Y p-sites” (representing serine, threonine, and tyrosine phosphorylation sites) and “Others”, (b) A bar graph comparing the number of predicted phosphorylation sites in ordered and disordered regions of GPx1, (c) A disorder prediction plot for GPx1, indicating the propensity of different regions of the protein to be disordered, (d) A phosphorylation prediction graph, highlighting potential phosphorylation sites within the GPx1 sequence, (e) A ubiquitination prediction graph, indicating potential ubiquitination sites within the GPx1 sequence.
Allelic frequency and clinical significance of proposed mutants
To delve deeper into the allelic frequency of the proposed mutants, we utilized a genome aggregation database, gnomAD v4.1.0 (https://gnomad.broadinstitute.org/)84. This extensive database offers information on multiple populations, which might aid in improving our understanding of the frequency of suggested mutations in various geographical areas. From gnomAD results, L168Q was a most frequent mutation found in about 128 exomes and 14 genomes all over the world. The other two mutations, G75C and F167S, were less abundant and reported in only 3 exomes each. More relevant information about allelic frequency is provided in Table 7.
Additionally, a database using already reported cases and publishes researches, named ClinVar (https://www.ncbi.nlm.nih.gov/clinvar/)85, was utilized to check the clinical significance of the proposed mutants. Based on the already submitted information, ClinVar categorized all of the three mutants (G75C, F167S, L168Q) as highly significant for clinical profiles.
GPx1 gene-gene interaction
GPx1-related gene connections were predicted using GeneMANIA and STRING v11. It was found that GPx1 and SLD3 physically interact. GPx1 is involved in the expression of many genes, including YOR052C, GTT1, SYM1, SOL4, FMP33, FMP16, GRX1, PRX1, TSA2, AHP, and HMX1. GPx1 is genetically related to GPx2, PRX1, HMX1, COX8, AHP1, TSA2, and YOR052C. Moreover, GPx2, GTT1, GTT2, AHP1, GRX1, PRX1, YGR201C, and TSA2 are among the protein domains it shares. Based on the STRING predictions, a total score was also assigned to each gene. The results of GeneMANIA and STRING are shown in Fig. 12.
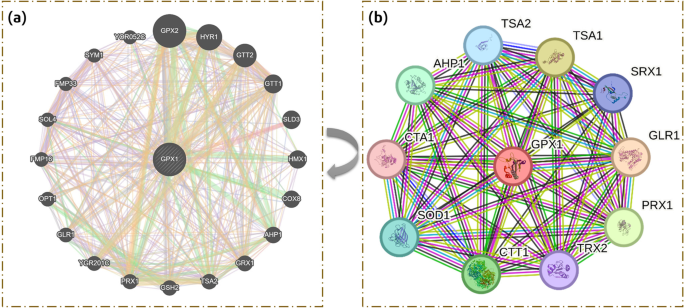
Depicts the predicted protein-protein interaction networks of GPx1 as assessed by two different tools; (a) The network generated by GeneMANIA highlights the functional associations and co-expression patterns of GPx1 with other genes in the human interactome (b) The network generated by STRING focuses on direct physical interactions between GPx1 and its predicted protein partners.